In today’s data-driven world, the integration of cutting-edge technologies like LLM knowledge graph drug purpose tools is reshaping healthcare and pharmaceutical industries. By leveraging the power of large language models (LLMs) and knowledge graphs, researchers and healthcare professionals can unlock unparalleled insights into drug mechanisms, efficacy, and potential uses. This comprehensive article delves into the role of LLM knowledge graph drug purpose, its applications, and its transformative impact on drug discovery and healthcare.
Table of Contents
Understanding LLM Knowledge Graphs and Drug Purpose
The synergy of large language models (LLMs) and knowledge graphs has created a potent framework for understanding complex medical data. LLM knowledge graph drug purpose refers to the application of these technologies to analyze, structure, and interpret information related to pharmaceuticals. While LLMs excel at natural language processing, knowledge graphs organize data into a structured format that connects entities, such as drugs, diseases, and genes.
By combining these technologies, LLM knowledge graph drug purpose provides actionable insights. For instance, this integration can predict new therapeutic uses for existing drugs or uncover potential side effects by analyzing vast datasets of medical literature, clinical trials, and biochemical pathways.
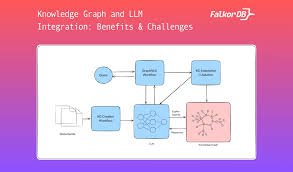
The Core Components of LLM Knowledge Graphs in Drug Research
The application of LLM knowledge graph drug purpose relies on three main components:
- Large Language Models (LLMs): LLMs like GPT-4 are trained on extensive datasets to understand and generate human-like text. They can interpret medical literature, identify patterns, and suggest hypotheses related to drug efficacy and safety.
- Knowledge Graphs: Knowledge graphs create a network of interconnected nodes that represent entities and their relationships. In the context of LLM knowledge graph drug purpose, these nodes might include drugs, diseases, genes, and molecular pathways.
- Integration Frameworks: Tools and frameworks integrate LLM outputs into knowledge graphs. This ensures that the information is both contextualized and actionable, enhancing drug discovery processes.
Applications of LLM Knowledge Graphs in Drug Discovery
The integration of LLM knowledge graph drug purpose offers diverse applications in pharmaceutical research and healthcare. These applications include:
Drug Repurposing The identification of new uses for existing drugs is a critical area of pharmaceutical research. By analyzing vast datasets, LLM knowledge graph drug purpose tools can identify potential off-label uses for medications, reducing the time and cost of drug development.
Adverse Drug Reaction Prediction Adverse drug reactions (ADRs) are a major concern in clinical practice. Knowledge graphs integrated with LLMs can predict potential ADRs by analyzing known drug interactions, genetic predispositions, and patient demographics.
Target Identification and Validation In the early stages of drug discovery, identifying and validating molecular targets is crucial. LLM knowledge graph drug purpose technologies streamline this process by connecting genetic and biochemical data to pinpoint viable targets for therapeutic intervention.
Personalized Medicine Personalized medicine aims to tailor treatments to individual patients based on their genetic makeup and medical history. Using LLM knowledge graph drug purpose, healthcare providers can identify the most effective therapies for each patient, minimizing side effects and improving outcomes.
Enhancing Clinical Trials Clinical trial design and recruitment can be optimized using LLM knowledge graph drug purpose systems. These tools can identify suitable patient populations, predict trial outcomes, and highlight potential risks.
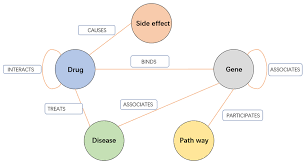
How LLM Knowledge Graph Drug Purpose Works
The process of implementing LLM knowledge graph drug purpose involves several steps:
- Data Collection: Data from various sources, such as medical journals, clinical trial databases, and genomic studies, is gathered.
- Natural Language Processing: LLMs analyze and interpret textual data, identifying key concepts and relationships.
- Graph Construction: The extracted information is structured into a knowledge graph, with nodes representing entities (e.g., drugs, diseases) and edges denoting relationships.
- Query and Analysis: Researchers query the knowledge graph to uncover insights. For example, they might ask how a specific drug interacts with a particular protein linked to a disease.
- Validation: Hypotheses generated by the system are validated through laboratory experiments or clinical trials.
Benefits of LLM Knowledge Graphs in Healthcare
The adoption of LLM knowledge graph drug purpose technologies offers numerous benefits, including:
- Enhanced Data Interpretation: LLMs can process vast volumes of unstructured data, extracting meaningful insights that would be impossible for humans to analyze manually.
- Accelerated Drug Discovery: By automating data analysis, these tools reduce the time required for drug discovery and development.
- Cost Efficiency: Identifying drug repurposing opportunities and optimizing clinical trials can significantly cut costs in the pharmaceutical industry.
- Improved Patient Outcomes: Personalized medicine and ADR prediction enhance patient safety and treatment efficacy.
Challenges in Implementing LLM Knowledge Graphs
Despite their potential, LLM knowledge graph drug purpose technologies face several challenges:
- Data Quality and Integration: Ensuring the quality and consistency of data from diverse sources is critical for building reliable knowledge graphs.
- Scalability: Handling large-scale datasets and maintaining graph performance can be technically demanding.
- Interpretability: Making the outputs of LLMs and knowledge graphs interpretable for non-expert users remains a challenge.
- Ethical and Regulatory Concerns: Data privacy and compliance with regulations, such as GDPR, are vital when working with sensitive medical information.
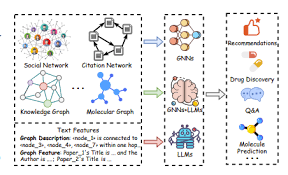
Future Directions of LLM Knowledge Graph Drug Purpose
The future of LLM knowledge graph drug purpose looks promising, with advancements in technology and increasing adoption across the healthcare sector. Key trends include:
- Integration with AI and Machine Learning: Combining knowledge graphs with advanced AI algorithms will further enhance predictive capabilities and decision-making.
- Real-Time Analytics: Real-time updates to knowledge graphs will enable dynamic insights, particularly useful in rapidly evolving fields like infectious disease management.
- Collaboration and Open Data Initiatives: Collaborative platforms and open data sharing will foster innovation and improve the accuracy of LLM knowledge graph drug purpose systems.
- Expansion into Global Health: These tools can play a significant role in addressing global health challenges by identifying cost-effective treatments for diseases prevalent in low-resource settings.
Case Studies Highlighting the Impact of LLM Knowledge Graph Drug Purpose
- COVID-19 Drug Discovery: During the pandemic, researchers used LLM knowledge graph drug purpose tools to identify existing drugs with potential efficacy against the SARS-CoV-2 virus. This accelerated the search for treatment options.
- Cancer Research: Knowledge graphs integrated with LLMs have been used to discover novel biomarkers and drug combinations for cancer therapies, improving precision medicine approaches.
- Rare Diseases: For rare diseases, where data is often sparse, LLM knowledge graph drug purpose technologies help identify potential therapeutic pathways by linking fragmented information.
Also Read: money6x.com Save Money: The Ultimate Guide to Smart Savings
Conclusion
The integration of LLM knowledge graph drug purpose tools is revolutionizing the healthcare and pharmaceutical industries. By combining the natural language processing capabilities of LLMs with the structured insights of knowledge graphs, these technologies are enabling faster, more efficient drug discovery and personalized treatment plans. Despite challenges, the ongoing advancements and growing adoption of these systems promise a future where healthcare is more precise, efficient, and patient-centered.